Predicting Transplant Rejection with AI
Illustration Mustafahacalak
A team of researchers from Emory, Case Western Reserve, and the University of Pennsylvania developed artificial intelligence tools to examine cardiac biopsy images to improve the prediction of rejection.
Clinicians now rely on histologic grading of cardiac biopsies to diagnose rejection, but the current criteria is vague and lacks diagnostic accuracy.
These limitations subject patients to considerable risk of receiving excessive or inadequate treatment.
The research team created a new method for automated, comprehensive analysis of heart biopsy images, the Cardiac Allograft Rejection Evaluator (CARE).
Using AI tools, CARE extracts features associated with the shape, texture and spatial architecture of muscle cells, immune cells, and stromal fiber in heart tissue specimen images to predict rejection outcomes for heart transplant patients.
“This facilitates the use of more aggressive treatments for those in need, leading to more effective prevention of heart transplant failure,” says researcher Sara Arabyarmohammadi of Case Western.
A study of 2,900 patients recently published in Circulation: Heart Failure showed that the CARE model, optimized to predict cardiac rejection severity, was far better at assessing a patient’s clinical outcome.
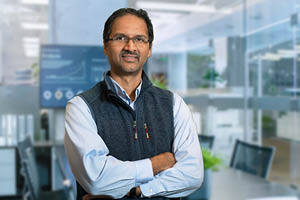
Anant Madabhushi, executive director of AI.Health at Emory